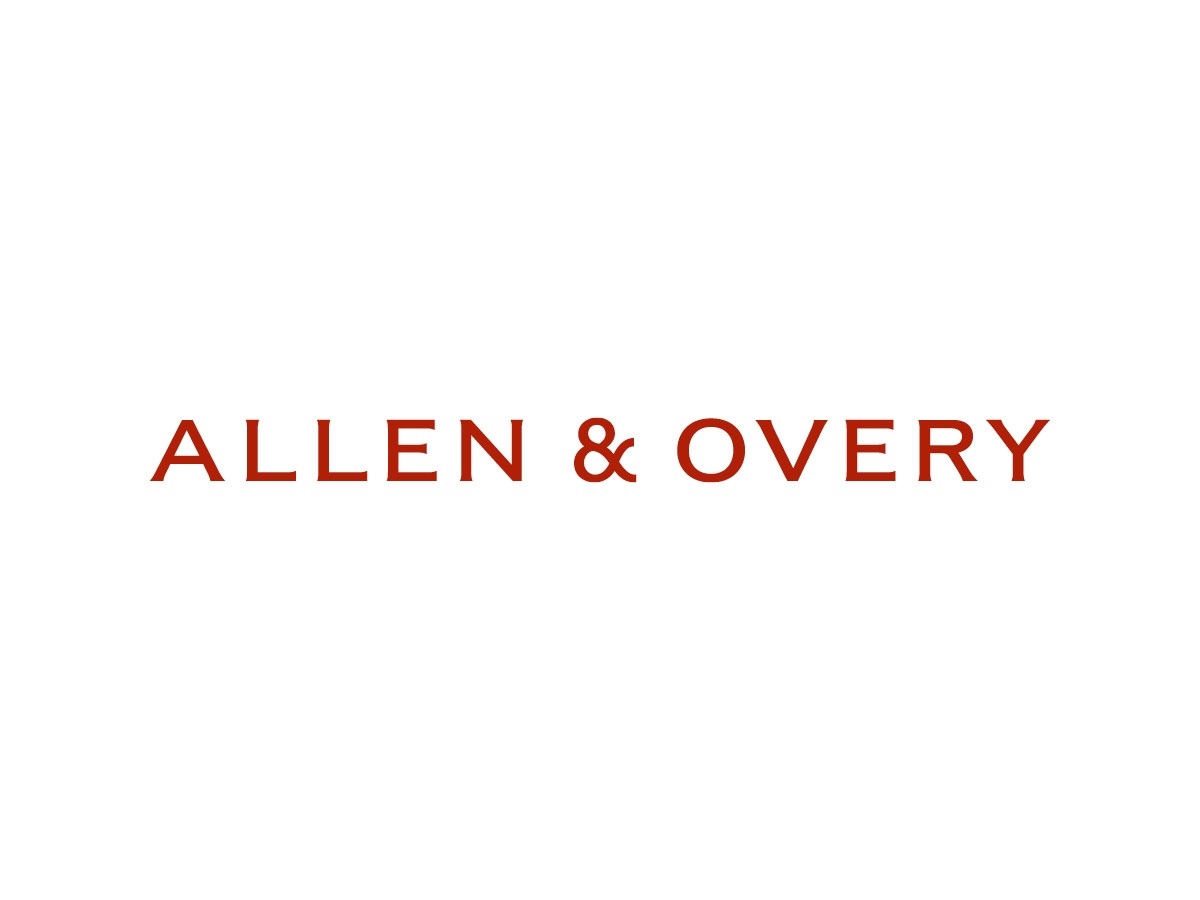
Artificial Intelligence in insurance – innovating in a world of increased regulation | Allen & Overy LLP – JDSupra
The potential benefits of deploying artificial intelligence (AI) (and in particular, machine learning (ML) techniques) within the insurance industry have been the subject of much market discussion and increased focus over recent years. Whereas attention was initially turned towards retail fraud detection software, clear use cases are now emerging throughout the insurance value chain across consumer and commercial lines, with the potential to improve the customer experience, facilitate better underwriting and portfolio risk management and create efficiencies in back-office operations.
While AI use cases currently in live, widespread deployment within the insurance industry remain (for the most part) relatively simple in nature, board level interest in, and the rate of adoption of, AI is increasing. Most incumbent firms have begun to dip a proverbial toe into the water of AI, with a view to identifying the “low hanging fruit” (in terms of deploying AI to maximise efficiency gains in areas of perceived lower regulatory risk) while they seek to understand the underlying technologies and their significant implications for operating insurance business in the future. Against this backdrop, the potential for regulatory lag has emerged in relation to the approach to the regulation of AI and the extent to which existing regulatory frameworks remain fit for purpose in the context of these developing technologies.
This note provides an overview of the status of adoption of AI within the insurance industry and the potential legal, regulatory and commercial challenges this exciting technology represents. It also draws from guidance published by financial services regulators to date to provide practical guidance to firms to identify features that may represent a heightened risk profile as use cases become increasingly complex, and develop risk mitigation strategies to assist those operating in this space to navigate the regulatory uncertainty, so as to facilitate innovation and expedite the adoption of AI within the sector.
A useful glossary of technical terms used in this note can be found in the Alan Turing Institute paper on “AI in Financial Services”.
How is AI being deployed within the insurance industry?
In its paper on “AI in Financial Services” commissioned by the UK Financial Conduct Authority, the Alan Turing Institute identified three key areas of recent innovation that have combined to facilitate the acceleration in deployment of AI within the financial services sector:
EIOPA’s paper “Artificial Intelligence Governance Principles: towards Ethical and Trustworthy Artificial Intelligence in the European Insurance Sector” outlined its findings on the proliferation of the use of AI across all parts of the insurance value chain, alongside anticipated AI use cases within the insurance industry and associated areas of regulatory concern. Key amongst these is the use of AI in underwriting and pricing, portfolio risk management across the existing book and on the retail side in particular, claims notification and fraud detection.
Given the anticipated significance of the application of ML to activities within the insurance value chain, the majority of the discussion in this note refers principally to AI use cases incorporating ML techniques.
Legal, regulatory and commercial challenges
There are various well documented legal, regulatory and commercial pitfalls when it comes to deploying AI in insurance settings. While many regulatory bodies worldwide have been specifically tasked with facilitating technological innovation within their respective spheres and certain jurisdictions (including the UK) have adopted national strategies for the growth of AI development, there is a clear acknowledgment that existing legal and regulatory frameworks will likely need to be revisited and adapted to address the potential risks and harms arising from the application of AI within financial services (and beyond).
In order to meet these competing objectives, regulators will need to address certain gating questions arising in relation to the regulation of AI, which (in of themselves) reflect the multi-faceted legal and regulatory risks posed and underline the complexity of the challenge faced by regulators:
In its July 2022 policy paper on “Establishing a pro-innovation approach to regulating AI”, the UK government set out its intention to establish a set of non-statutory, cross-sectoral principles tailored to the distinct characteristics of AI, with regulators being asked to interpret, prioritise and implement these principles within their sectors and domains, all through a pro-innovation lens bearing in mind the importance of proportionality and adaptability. While this sounds great on paper, it is easy to see how challenging the task at hand is for the regulatory community. The scale of the task and the pace of technological development combined with the limited resources undoubtedly increases the scope for regulatory lag.
In the meantime, understanding, identifying and managing these legal, regulatory and commercial challenges will be critical for any industry participant developing or using (directly, or via its suppliers or subcontractors) AI (and in particular, ML) as part of its business, as well as any institutional investor evaluating opportunities within this space:
Risk assessment factors
However regulation in this space develops, proportionality will be the guiding principle. Indeed, in a statement delivered in February 2022 entitled “AI governance: Ensuring a trusted and financially inclusive insurance sector”, EIOPA expressed support for the “risk-based approach” adopted by the European Commission in the Draft EU AI Reg, noting that “not all AI systems pose the same opportunities and risks and hence the need for proportionality”. Similarly, the UK Prudential Regulation Authority noted in its October 2022 discussion paper on “Artificial Intelligence and Machine Learning” that “a proportionate approach is critical to supporting the safe and responsible adoption of AI and other technologies across UK financial services”.
When embarking on a project or transaction involving the use of AI within the insurance industry (particularly those incorporating ML techniques), legal and compliance teams will need to be alive to features that may represent a heightened risk profile and necessitate a proportionately greater level of diligence and/or governance and monitoring. While risk factors will be specific to individual use cases and should be assessed in the relevant context, considerations in relation to key areas of risk at the various stages of the AI lifecycle are set out below:
AI supply chain (e.g. in circumstances where: (i) the development of an AI tool for use within an insurance business is being outsourced; (ii) insurance business activities are being outsourced to a third party provider that utilises AI tools; or (iii) insurance business activities are undertaken in-house using AI tools provided by a third party)
System design
System monitoring
System performance
Risk mitigation strategies
To the extent not already in place, firms should ensure that appropriate frameworks are implemented to both mitigate the risks arising from the development and deployment of AI within its insurance business and manage them in a proportionate manner. Risk mitigation strategies include:
board / senior management oversight:
internal governance frameworks – internal governance frameworks and controls to be implemented covering:
This content was originally published here.